8 Unit Testing Examples for Better Code Quality
Most teams treat unit tests like a safety net but that net has holes. Hitting 100% test coverage looks impressive in CI dashboards, but it often hides the truth: you’re not testing the right things. Minor changes slip through and break production without triggering a single failure.
A 2025 analysis of over 200 million lines of code committed in 2024 found that, for the first time, 'Copy/Pasted' code lines exceeded 'Moved' (refactored) lines, coinciding with an eight-fold increase in duplicated code blocks. It’s a sign that many teams are prioritizing output over quality, with duplicated logic slipping through weak tests that can’t handle change.
This guide isn’t about coverage for the sake of metrics. It’s about writing tests that catch real bugs, survive rewrites, and give you confidence when it’s time to ship. We’ve included 8 examples you can drop into your own stack—patterns that prioritize signal over noise, so you stop wasting time on brittle mocks and start building with speed.
Table of Contents
There’s no shortage of unit tests in most modern codebases. But useful ones are rare. Coverage targets are hit, CI pipelines go green, but regressions still ship. That’s usually because the wrong things are being tested. Clean, concise tests are part of a productive workflow.
Bad unit tests share patterns:
- They mock too much, testing implementation rather than behavior.
- They pass when the app is broken, because nothing meaningful was asserted.
- They break during harmless refactors, adding friction instead of safety.
Useful unit tests do the opposite:
- They lock down critical logic, not trivial getters or framework behavior.
- They cover edge cases, not just the happy path.
- They stay stable across internal changes because they test what the code does, not how it's written.
If your test suite constantly breaks when you rename a class or swap a library, it’s not protecting you—it’s slowing you down. This guide covers 8 patterns that flip that dynamic: tests that survive real-world development and surface actual issues before they hit prod.
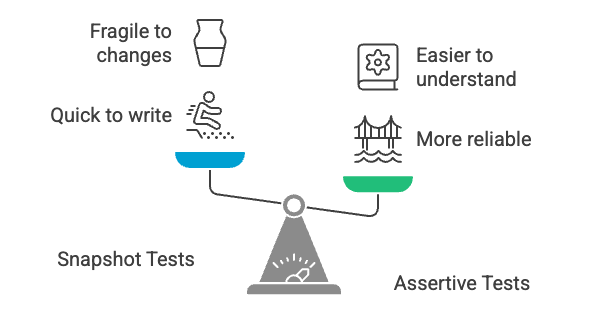
Snapshot vs Assertive Tests.
Snapshot tests are quick to write but easy to misuse. If you're testing components with dynamic content, frequent layout tweaks, or even just evolving prop structures, snapshots become cluttered. They catch changes, not necessarily bugs. That makes them great for catching unexpected diffs in static output—but terrible for behavior validation.
Assertive tests are more work up front, but they fail when it actually matters. They force you to decide what correctness means. Is the button there? Is the text right? Was the callback triggered? These are the questions users care about—your tests should care too.
Use snapshot tests only when:
- The component is mostly static (e.g., icons, layout scaffolds, boilerplate content).
- You’re locking down a structure that rarely changes and should never change silently.
Use assertive tests when:
- The component has conditional rendering, side effects, or user interaction.
- You need clarity in failures. Snapshot diffs don’t explain why something broke.
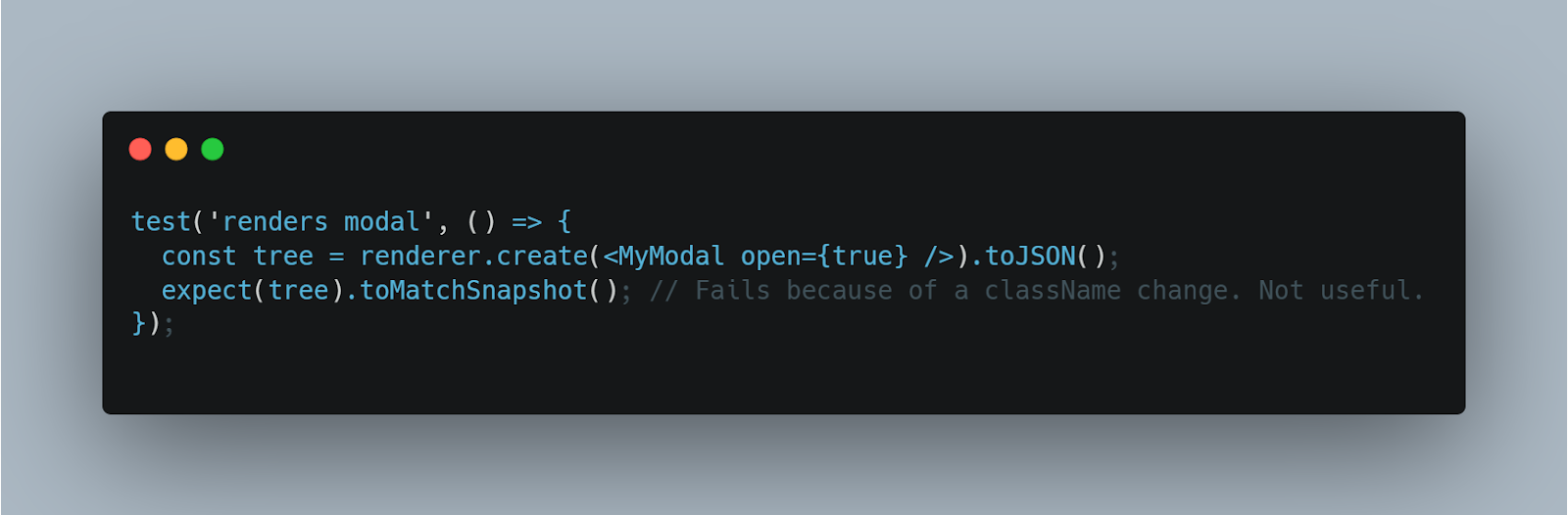
Bad: snapshot test on dynamic UI. A unit testing example in practice.
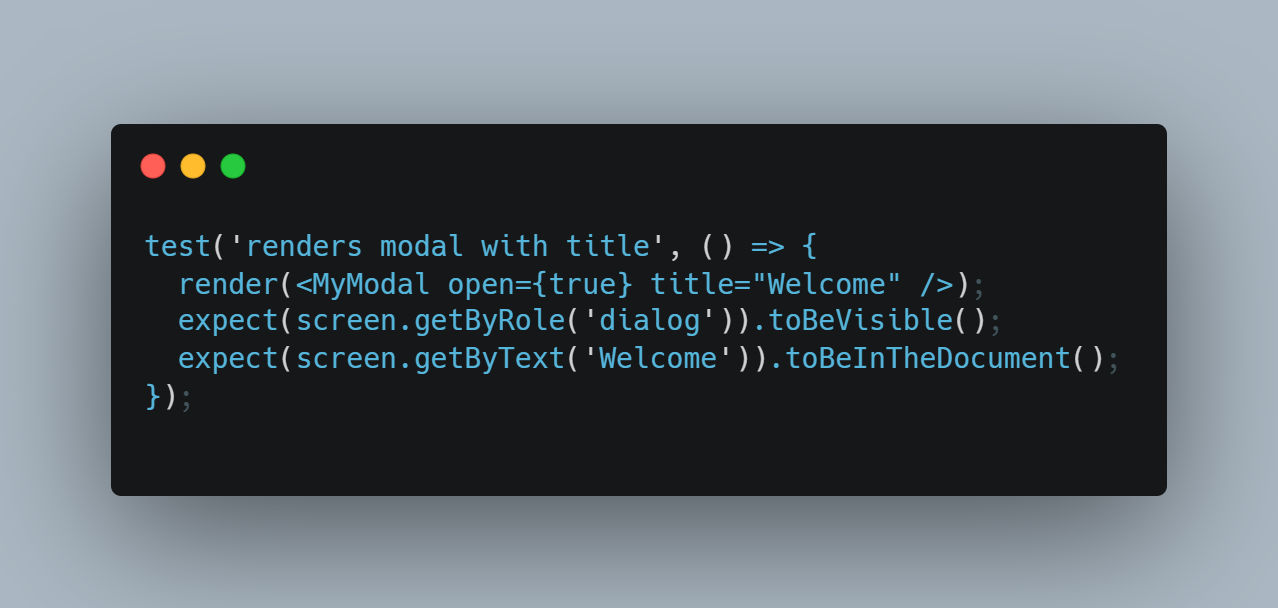
Good: assertive test on visible behavior.
These types of unit testing examples show why asserting behavior, not structure, matters.
Mocking is supposed to make your tests faster and more predictable. The following unit testing examples show what happens when mocks go too far. But the second you start mocking everything, your tests stop validating real behavior. Now you're just testing your own assumptions.
Here’s the best practice: mock the edge, not the core.
That means you mock what you don’t control (external APIs, cloud services), but you let your application logic run for real. The point is to isolate instability—not to pretend you’re writing tests when you’re actually hardcoding a successful outcome.
JavaScript – Mocking axios responsibly example:

Anti-pattern: mocking axios and faking internal logic. Now your test can’t fail, even if the UI breaks.
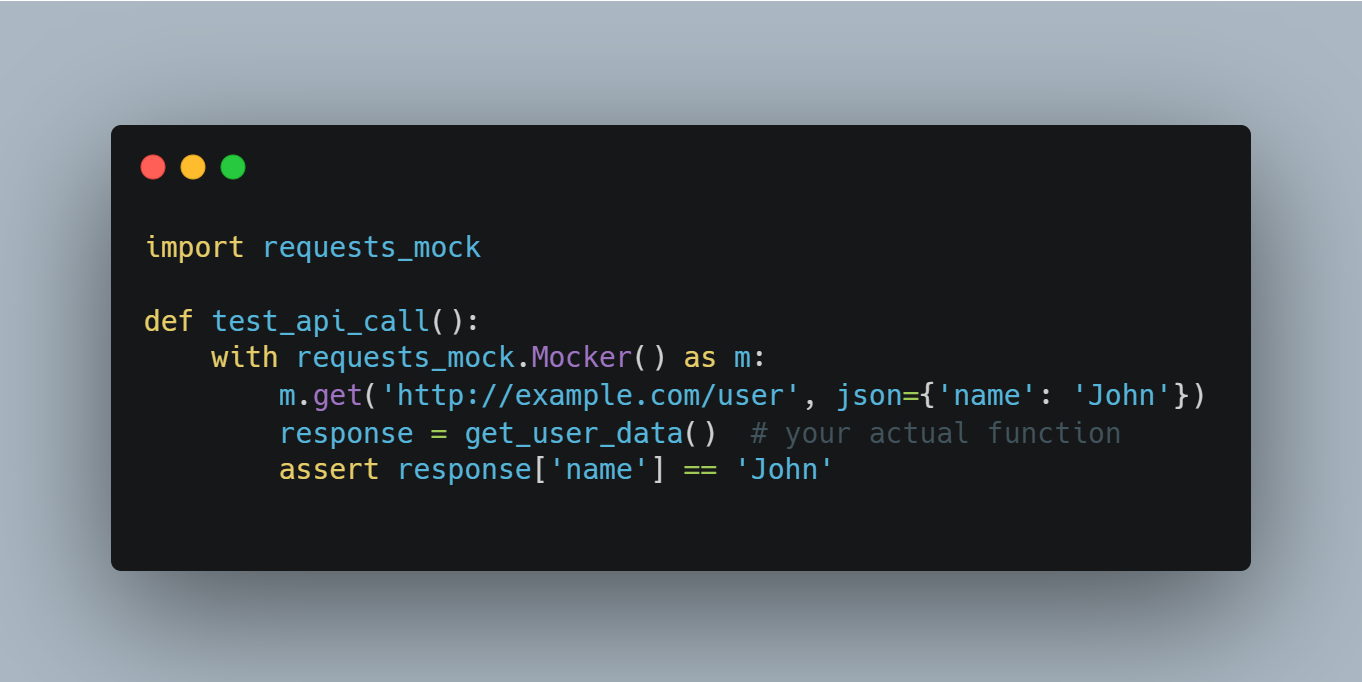
Python – Using requests-mock for isolation
What Not to Do:
- Don’t mock internal functions just to make tests pass faster.
- Don’t simulate impossible scenarios (like 200 OK with garbage data) unless you’re explicitly testing edge cases.
- Don’t mock APIs you fully control (like internal microservices in integration tests)—use test containers or local servers when possible, and if those environments touch real services or data, be sure to secure them properly to prevent data leaks and breaches.
Instead, ask:
- Would this test still catch a real bug?
- Does the mock reflect what the real API actually returns?
- If the API changes, will this test alert me?
If the answer is no, your mock is lying. Strip it back.
Some of the most overlooked unit testing examples involve redundant tests that could be parameterized. If you’re writing the same test five times with different values, you’re doing it wrong.
Parameterized tests let you define one assertion, then run it across dozens of inputs—without bloating your suite or hiding critical cases in repetitive boilerplate. But it’s not just about DRY. Used well, parameterized tests force you to think about the shape of your data and where edge cases live.
Use parameterized test for edge cases when:
- You’re testing pure functions or logic with clear input/output mappings.
- You need to validate boundary conditions—e.g., 0, null, empty strings, invalid types.
- You want fewer test names with more test depth.
Don’t use them when:
- Each case needs different setup/teardown logic.
- The test fails and gives you useless output like "expected false to be true" without context. If the failure message isn’t actionable, split it out.
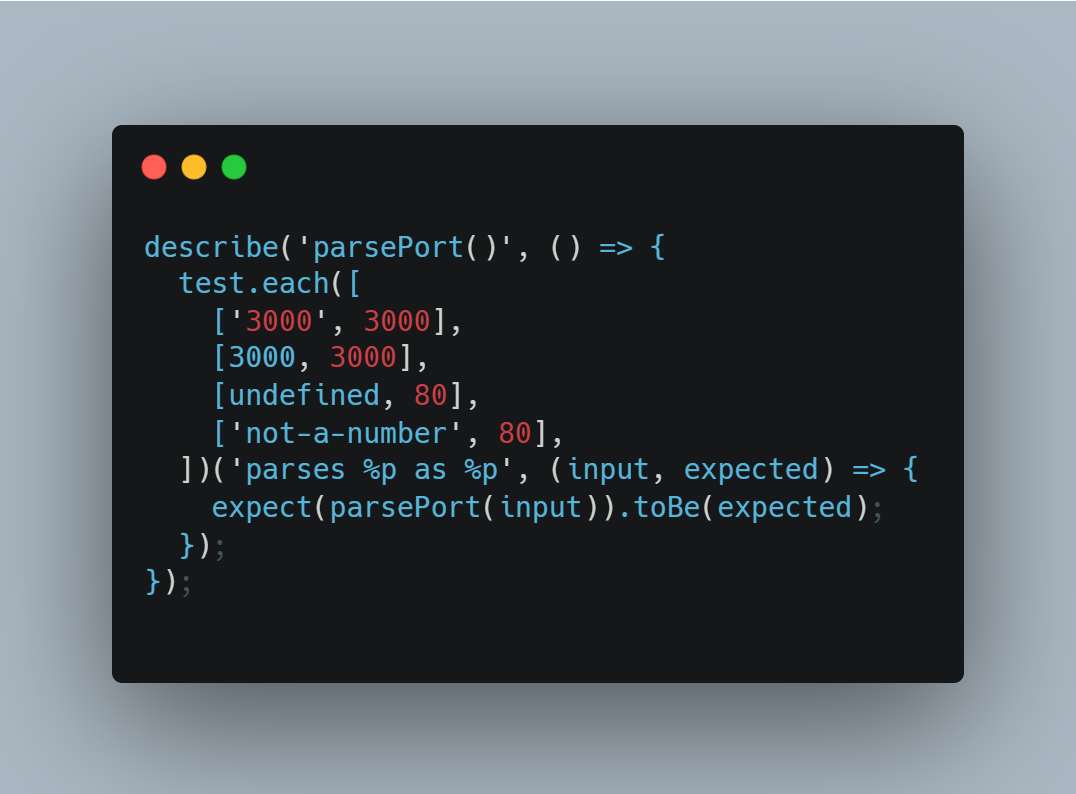
Example (JavaScript + Jest)

Example (Python + pytest)
Most teams write tests to prove the code works. You should also write tests to prove it fails—intentionally, loudly, and at the right time. How? By intentionally introducing a bug or changing something to confirm that your test catches the issue.
These are red path tests: inputs that should be rejected, flows that should never succeed, and edge cases where the system must throw, not crash silently.
What to cover in red tests:
- Invalid user input (e.g. malformed emails, nulls, types that don’t belong)
- Unauthorized access attempts
- Timeouts or upstream service failures
- Business logic constraints (e.g. “you can’t refund a refunded order”)
Red tests double as living documentation - which is a key part of strong security policies. They show future devs exactly what inputs are out of bounds, and what kind of errors to expect. That makes them essential for debugging, onboarding, and API reliability.
Off-by-one errors are stealth bugs. They pass casual testing, sneak past code review, and show up in prod when a user hits exactly 0, 1, or max + 1.
These aren’t just fencepost errors in loops—they hit everywhere:
- Passwords that reject valid input
- APIs that include too much or too little data
- Pagination that skips or duplicates records
- Length or range validations that allow just-out-of-bounds input
Most bugs live on the edges of your input range and not the middle.
Tests should give you confidence to change your code—not punish you for doing it.
If your test suite breaks every time you rename a variable, extract a method, or reorder logic, then it's testing how your code works instead of what it’s supposed to do. That’s just a false safety net.
Refactor-proof tests focus on outputs and public interfaces. They validate results that users or systems actually depend on—not the steps your code takes to get there. This means:
- Don’t test private methods or internal helpers unless they are public contracts.
- Avoid asserting intermediate values that might change during optimization.
- Stick to validating what the system does, not how it does it.
The test should stay green if the behavior hasn’t changed—even if the code behind it looks completely different. That’s what makes a test valuable - that it doesn’t fail for irrelevant reasons.
Test speed is a design problem. If your test suite takes 5+ seconds, it won’t run often enough to be useful—especially during feature development or debugging.
You don’t need to benchmark every test, but sub-second execution should be a hard boundary for unit-level tests. Integration and E2E tests have different expectations—but unit tests are supposed to be cheap. If they’re not, you’re doing too much.
What slows tests down:
- Hitting the real database instead of mocking at the boundary.
- Waiting on network requests or background jobs to complete.
- Spinning up full app contexts instead of testing modules or pure functions.
- Rebuilding state on every run instead of using test fixtures or factories correctly.
Tests should isolate logic. If you're validating that a function returns the right output, you don’t need to boot the whole system. Mock external dependencies once, return known values, and assert behavior.
It’s not just about mocking everything either. You should mock intentionally—at the system edges, not inside your core. Don’t mock internal functions just to cut runtime; cut the code under test to the smallest meaningful slice.
Code coverage tells you which lines ran—not whether they were tested.
You can have 100% coverage and still miss critical bugs. That happens when tests execute code without asserting anything meaningful. If the test doesn’t validate behavior, it’s just noise.
A function that runs without throwing is not the same as a function that works, especially in Python.
This is common in autogenerated or checklist-driven test suites. A test calls a method, maybe passes some input, but never checks the output. It shows up as “covered” but doesn’t prove correctness. That’s how you end up with green pipelines and broken features.
You’re not trying to verify that code exists. You’re verifying that it does the right thing, under the right conditions, with the expected result.
What to check for:
- Does the test assert the exact outcome you care about?
- Are failure paths tested with negative assertions?
- Is behavior under edge input conditions being validated?
You don’t need more tests—you need better ones. The right unit testing examples make all the difference. The kind that catch real bugs, support safe refactors, and don’t break when the code improves.
This guide gave you unit testing examples that work in the real world. If your current tests are flaky, fragile, or faking it, it’s time to raise the bar.
EarlyAI is an agentic AI—tools that make decisions, not suggestions. It understands intent, context, and structure, and produces tests that are fast, resilient, and behavior-driven by default. If you’re still dealing with flaky snapshots, useless mocks, or untested failure paths, EarlyAI can step in and fix that autonomously.
Pick one of your tests. Is it doing its job? If not, either fix it—or let EarlyAI write the one that should have been there in the first place.
Book a demo today and see unit testing examples in action.